Lorem ipsum dolor sit amet, consectetur adipiscing elit. Ut elit tellus, luctus nec ullamcorper mattis, pulvinar dapibus leo.
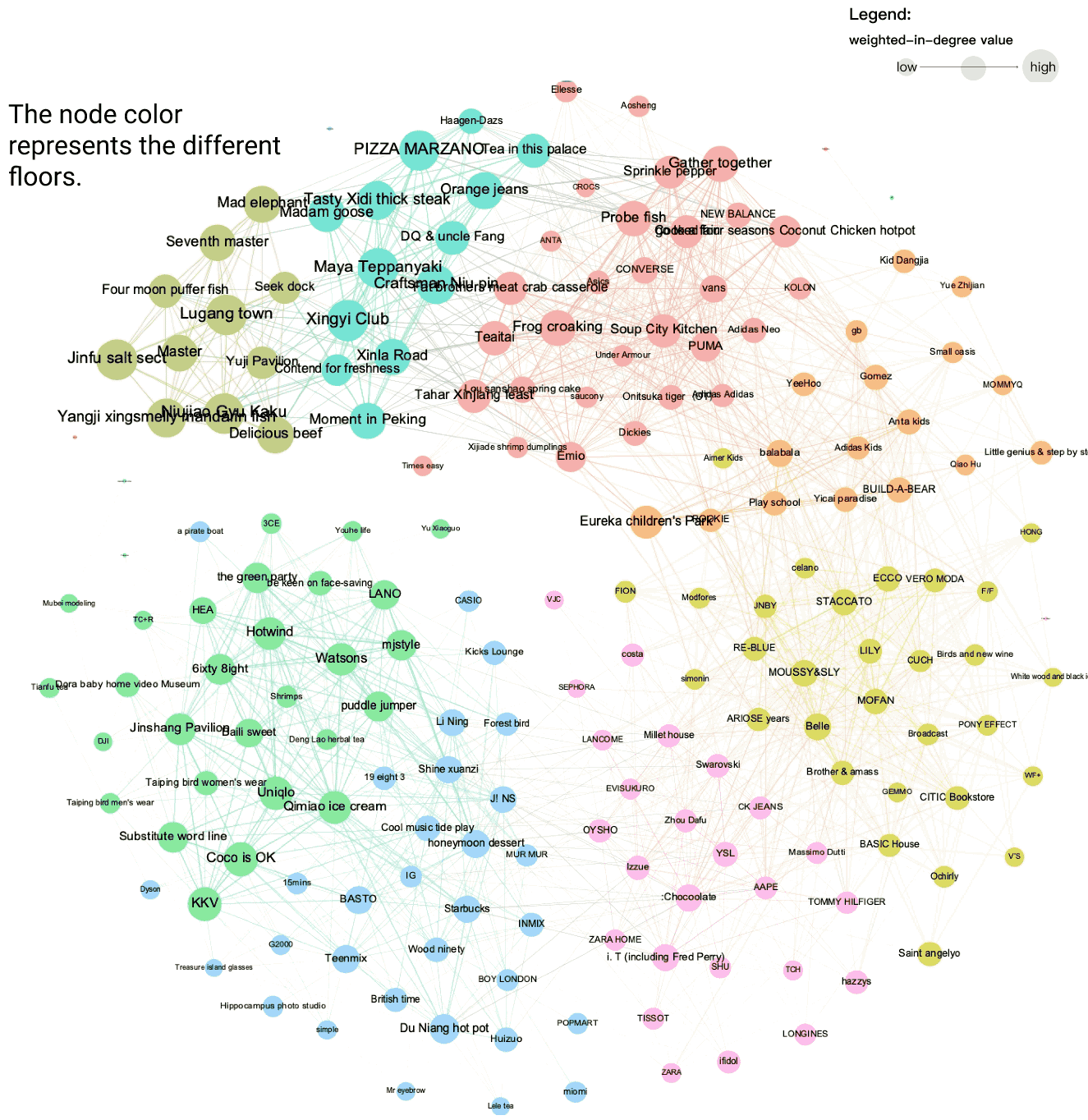
A well-designed tenant mix in the shopping mall will generate positive spillover effects among tenants, which will bring extra foot traffic and sales to tenants and improve the overall economic performance of the shopping mall. Thus, a clear measurement of the intensity and direction of retail spillover effects are significantly important to the success of shopping mall management and operation. The spillover effects of tenant mix at the mall level have been well articulated by extant studies, but very few studies have tried to quantify the spillover effects among tenants inside the shopping mall, which is mainly due to the absence of granular data in measuring the tenant economic performance and consumers’ behaviors. As more and more shopping malls are seeking digital transformations, new granular datasets, such as detailed tenant sales data and consumer foot traffic data, are available. This study aims to fill the gap in quantifying retail spillover effects at the tenant level with the daily tenant sales data and consumer trajectories data from a study shopping mall in China. This study will answer the research questions below:
- What is the source of the spillovers? spatial proximity, price similarities, tenant type complementarity?
- How to precisely quantify the intensity, direction and location tenant-level spillovers?
- Are sales spillovers and footfall spillovers linked?
This study develops a threefold analysis framework to quantify the spillover effects from the sales and footfall perspectives. The sales spillover analysis result shows that retail spillovers are mainly generated from the spatial and type proximity in the study shopping mall and reveals that spillover effects mainly concentrate in the restaurant agglomeration and anchor store area. The proceeding footfall analysis will allow us directly measure the spillovers and reveal the links between sales and footfall spillovers. This new data-driven analysis pipeline is applicable to analyze other shopping malls, or to be developed into management products for the real estate industry.
1. Overall tenant spillover in terms of sales
We construct three regression models to examine the existence of spillover effects at the shopping mall level with tenant daily sales data. The baseline model is the classic spatial lag regression. The multiple lag regression model includes price, popularity and type similarity matrices. The cumulative spatial lag regression further examines spatial attenuation of spillover effects.
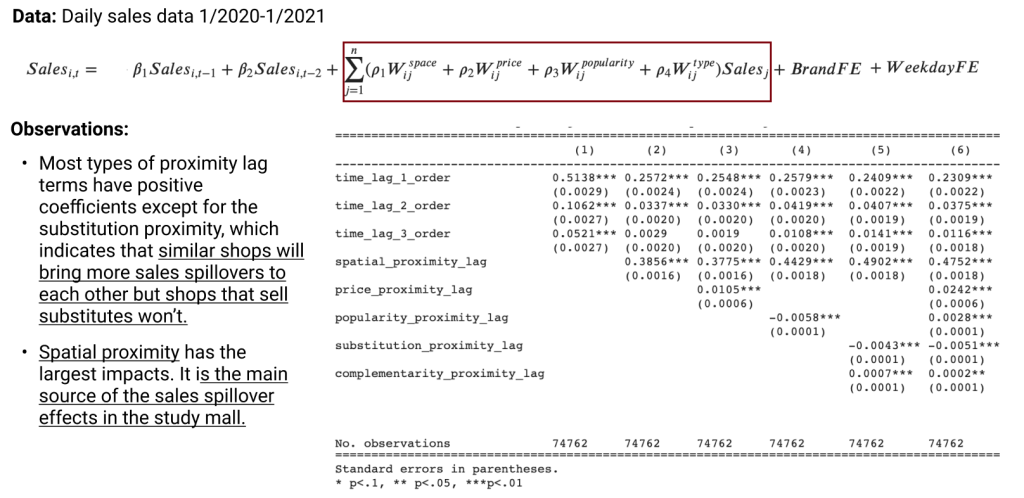
2. Tenant level spillover in terms of sales
Since sales and visitor data is non-stationary and have strong weekly seasonality, we use a seasonal differencing to make the time series stationary. Two stores which are adjacent to another store can be correlated, leading to the inference of spurious spillover effects. To solve this, many studies in the financial and genomics field use partial correlation. The partial correlation measures the degree of association between two random variables, with the effect of a set of controlling random variables removed. We calculate the partial correlation matrix among shops and use it as the link weight matrix to construct the sales and visitor count relatedness networks.
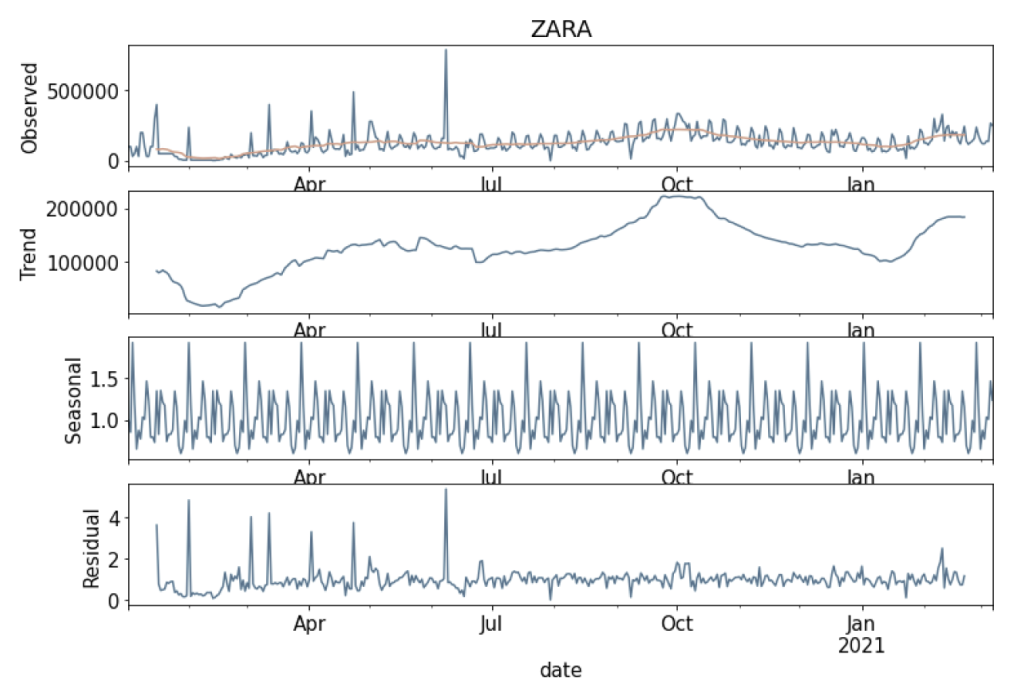
The graph shows clusters of tenants have very strong spillover effects among each other:
1) The restaurants and the entertainment tenants on the 5th and 6th floor;
2)The restaurants on the 4th floor;
3) The fast fashion and beauty brands near the subway entrance on the basement floors.
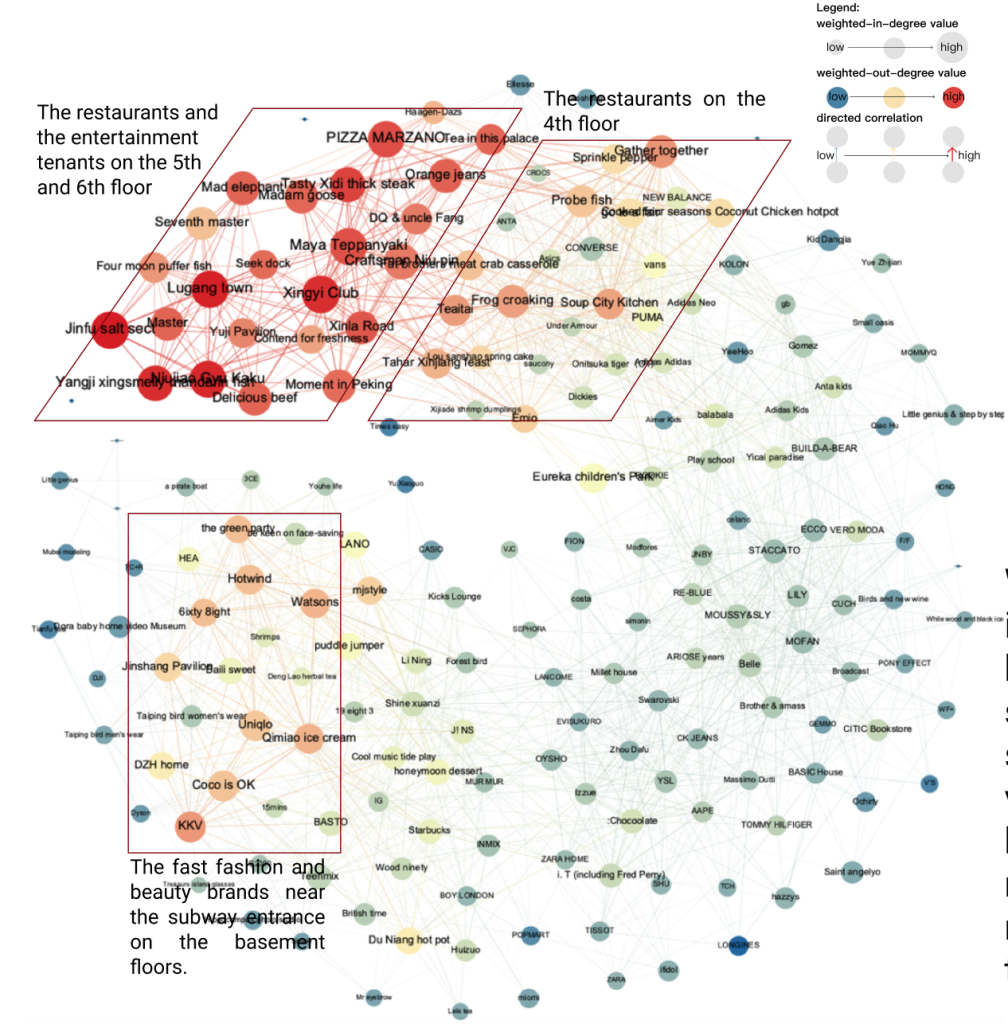
We use the average weighted inwards correlation to indicate how likely a tenant benefits from spillover generated by the surrounding tenants and vice versa. The majority of fitness and beauty salon tenants in the study mall are isolators, while most restaurants and fast fashion tenants are in-out balanced.
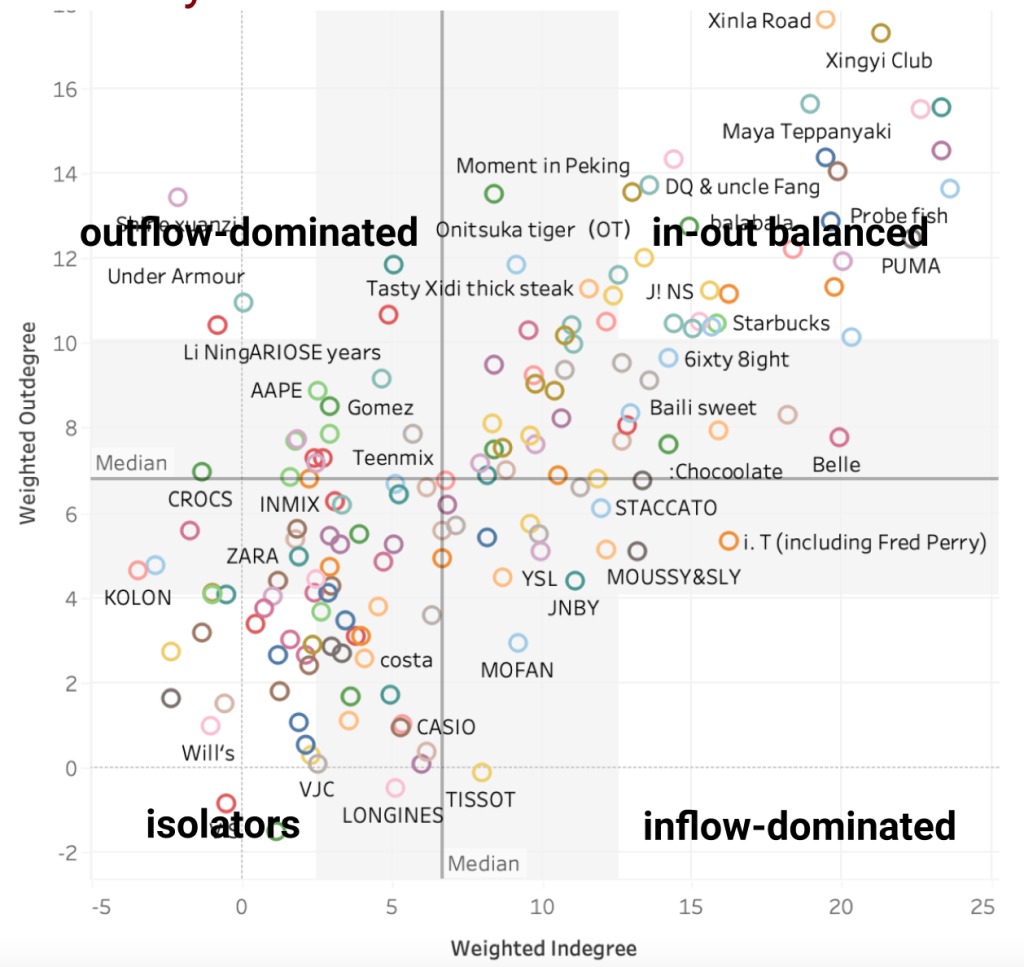
3. Tenant level spillover in terms of footfall (in progress)
Many existing studies have pointed out that consumers’ demand for shopping or visiting various stores during a shopping trip is the root driver behind the spillover effects. Thus, we will construct the footfall sharing network among stores with the same methodology that is used in the sales spillover effect. Then we further measure the alignment of the two networks with Known Node-Correspondence (KNC) methods to see whether the sales impacts between stores are related to the foot traffic impacts.
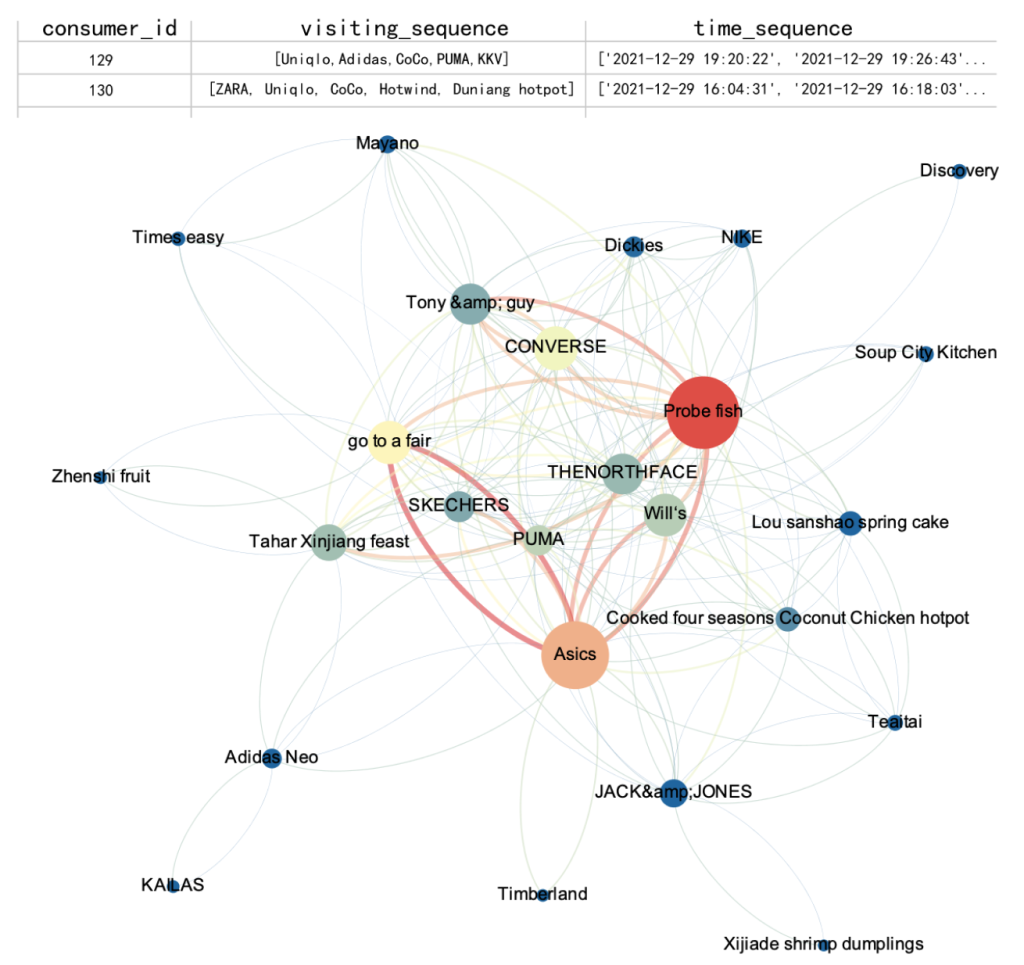